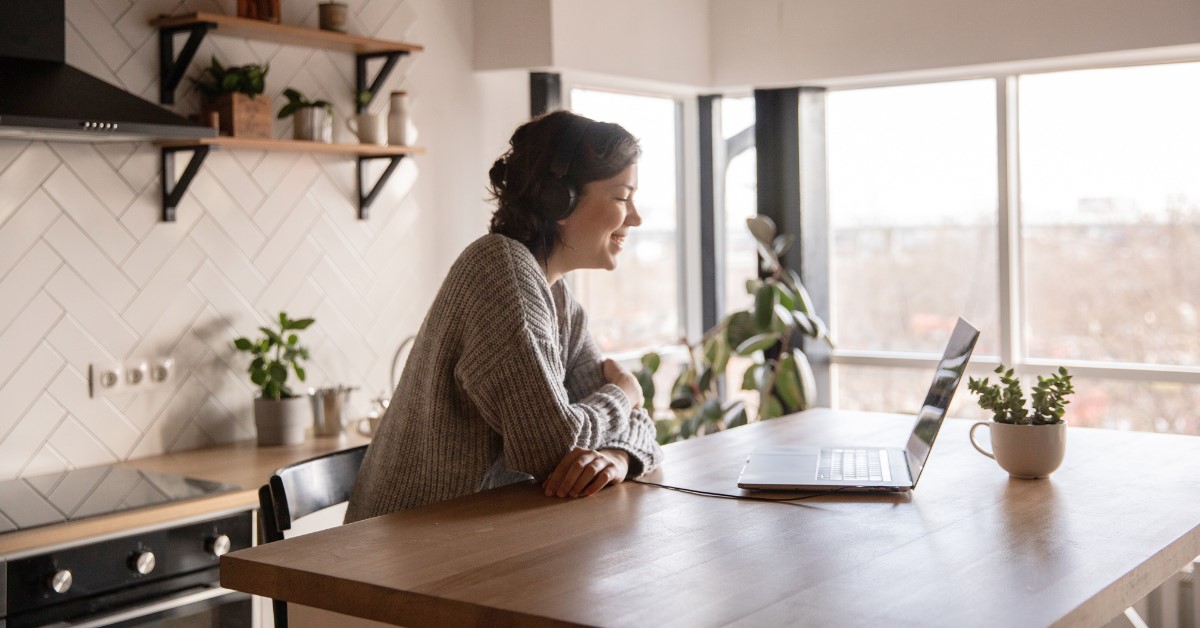
Implicit bias has been a problem within recruitment for as long as the industry has existed. AI gives us the chance to almost entirely remove or worsen these, depending on the ethical rigour of an algorithm.
Whether or not AI holds implicit bias has everything to do with the humans it interacts with.
From a training perspective, the data collection and machine learning methodologies are susceptible to prejudice. If biases do find their way into an AI algorithm, they are almost always amplified. This is hugely problematic. If the AI is no longer impartial, neither is the recruitment process.
Biases in Recruitment
Confirmation Bias
When we hear a piece of information that fits in with our beliefs, we are more likely to believe and retain it. In recruitment, this hinders the search because it prevents us from thinking outside the box and giving diverse talent a chance.
Confirmation bias causes us to actively look for certain traits, rather than assessing candidates objectively.
Name Bias
As a name is the first piece of information we’re given about a candidate, it’s human nature to base our initial judgement on it. But name bias often leads to other biases as we start to form an unsubstantiated opinion about someone’s background, qualifications or capabilities.
Name bias may lead to racial, gender and confirmation biases. We could even compare the candidate to people in our lives with the same name.
The Halo Effect
The Halo Effect explains why conventionally attractive people are likely to be judged as better, kinder, smarter and more successful. In recruitment, it may not be physical appearance, but one positive trait learned early on can influence our overall opinion of a candidate.
The opposite of The Halo Effect is The Horn Effect. One negative trait negatively influences the way a candidate is judged overall.
The Primacy and Recency Effects
Even the order we conduct our search can influence our perception of candidates.
When sifting through multiple CVs, conducting back-to-back interviews or scouring Sales Navigator lists, human recruiters are more likely to remember (and favour) candidates at the start (Primacy Effect) or end (Recency Effect) of their list.
Ethical Considerations When Using AI
AI can both remove and worsen biases in recruitment searches. Computers aren’t susceptible to the primacy and recency effects, for example. Despite potential benefits, there are ethical considerations to take into account.
Algorithmic Bias
Implicit biases in humans can be passed on to machine learning algorithms. Labelling bias, for example, occurs when humans labelling data early on in the training process let their implicit bias affect their labelling.
To find out more about how implicit bias sneaks into AI’s algorithms, check out How Problematic is Implicit Bias in Artificial Intelligence? In Orbis’ magazine: AI in Recruitment.
Accountability
When AI makes decisions, who is held accountable for the consequences? Poor hiring decisions or unethical interview practices may be the fault of the algorithm, but most clients want a human held responsible for mishaps.
The same goes for great work. Will AI receive praise and promotions?
Data Privacy and Consent
The machine learning process involves huge datasets. In recruitment, these datasets include confidential personal and business information.
While individuals and businesses may consent to their data being used to train AI models, it’s a grey area when that data is then used to analyse hiring decisions that affect them personally.
Ethical Use of Recruitment AI: Best Practice
Even the best recruiters have biases. It’s part of what makes us human. It is, therefore, up to us to ensure they don’t form part of our hiring decisions. To keep implicit bias out of an AI-driven search methodology, follow Orbis’ best practice tips:
- Keep humans involved: Humans should be making final decisions and overseeing the output of AI. A human should be responsible for every stage of the search, including decisions made by AI.
- Maintain diverse talent pools: Diverse talent pools are critical for keeping AI unprejudiced. Collect this data anonymously and raise diversity concerns immediately.
- Ensure training processes are unprejudiced: Ensure the data and human input used to train AI are unprejudiced. Collect wide, diverse data and educate trainers on implicit biases.
- Use objective, standardised criteria: Ensure all candidates are being judged against the same standardised criteria. It might be useful to perform these checks using AI before human judgement gets involved.
- Audit algorithms frequently: Regularly check and update algorithms to identify and remove biases. This includes checking your search methodology against legislative and ethical best practices.
- Obtain informed consent: Ensure candidate and company data is used only in the way the owner has consented. That includes follow-up projects and future algorithms. You should also tell candidates if AI is being used to make hiring decisions.
- Ask candidates for feedback: Ask candidates how they feel about the search process. Allow anonymous feedback to encourage honesty and reduce demand characteristics.
- Collaborate with industry bodies and D&I teams: Keep up with the latest industry best practices to use AI ethically. If possible, get involved in the decision-making process by participating in policymaking activities.
To learn more about using AI as part of your search methodology, download the Orbis magazine on AI’s impact on the recruitment industry. Included is a ChatGPT Cheat Sheet to help you make the most of the world’s favourite AI chatbot.